2019年1月27日 星期日
2019年1月26日 星期六
解安裝ubuntu 16.04本身內建python3.5
1.卸载python3.5
sudo apt-get remove python3.5
2.卸载python3.5以及它的依赖包
sudo apt-get remove --auto-remove python3.5
3.清除python3.5
要想清除python3.5的配置文件和数据文件,执行以下命令:
sudo apt-get purge python3.5
sudo apt-get purge --auto-remove python3.5
sudo apt-get remove python3.5
2.卸载python3.5以及它的依赖包
sudo apt-get remove --auto-remove python3.5
3.清除python3.5
要想清除python3.5的配置文件和数据文件,执行以下命令:
sudo apt-get purge python3.5
sudo apt-get purge --auto-remove python3.5
Error after upgrading pip: cannot import name 'main'
$ sudo python3 -m pip uninstall pip && sudo apt install python3-pip --reinstall
2019年1月25日 星期五
sudo: pip: command not found
Ubuntu系統下執行sudo pip install package-name出現 sudo: pip: command not found 的問題。原因是因為編譯sudo的時候加入了–with-secure-path 選項。
$ vi ~/.zshrc or vi ~/.bashrc
$ alias sudo='sudo env PATH=$PATH'
$ source ~/.zshrc or source ~/.bashrc
參考
https://blog.csdn.net/hhhuua/article/details/80995339
$ vi ~/.zshrc or vi ~/.bashrc
$ alias sudo='sudo env PATH=$PATH'
$ source ~/.zshrc or source ~/.bashrc
參考
https://blog.csdn.net/hhhuua/article/details/80995339
zsh: command not found: conda
$ vi ~/.zshrc
$ export PATH="/home/chiustin/anaconda3/bin:$PATH"
$ source ~/.zshrc
$ export PATH="/home/chiustin/anaconda3/bin:$PATH"
$ source ~/.zshrc
2019年1月23日 星期三
python判斷作業系統
$ import platform
$ sysstr = platform.system()
platform.system()會傳回 Windows、Linux、Darwin(Mac OS X)、Java(Jython) 等字串
$ sysstr = platform.system()
platform.system()會傳回 Windows、Linux、Darwin(Mac OS X)、Java(Jython) 等字串
參考
MAC在終端機執行開啟vmd
1.寫到
$ vi ~/.zshrc
alias vmd='/Applications/VMD\ 1.9.4.app/Contents/Resources/VMD.app/Contents/MacOS/VMD'
2.套用
$ exec $SHELL
3.執行vmd
$ vmd
參考
http://kanjulin.blogspot.com/2014/02/vmd-run-vmd-from-mac-terminal.html
http://macosxpostdoc.blogspot.com/2011/04/how-run-vmd-from-terminal-of-os-x.html
$ vi ~/.zshrc
alias vmd='/Applications/VMD\ 1.9.4.app/Contents/Resources/VMD.app/Contents/MacOS/VMD'
2.套用
$ exec $SHELL
3.執行vmd
$ vmd
參考
http://kanjulin.blogspot.com/2014/02/vmd-run-vmd-from-mac-terminal.html
http://macosxpostdoc.blogspot.com/2011/04/how-run-vmd-from-terminal-of-os-x.html
Ubuntu 18.04 單機安裝torque
雖然python可以批次化submit job,但如果job在跑的同時,有人利用ssh另外submit job,將會發生大災難,因此ubuntu 18.04可以安裝Torque避免悲劇發生。
2019年1月22日 星期二
2019年1月21日 星期一
Ubuntu 18.04終端機優化
有用過MacOSX都知道Oh My ZSH+iterm2優化終端機功能是多麼的強大,以下要介紹ubuntu18.04系統如何優化終端機,雖然無法在ubuntu安裝iterm2,但還是有其他套件可以取代MacOSX的iterm2喔。
vim indent
1. 多行縮排
按大V進入 -- VISUAL LINE -- 模式
選好要縮排的行之後按大於 ( > )
被選到的行就會縮排囉
2. 單行縮排
在一般模式下
游標在該行上,按兩下大於 ( >> )
那一行就會縮排了
3. 將大括弧的整個區塊縮排
先將游標移到括弧上,
按下大於,百分比 ( >% )
整個括弧區塊就會一起縮排囉
按大V進入 -- VISUAL LINE -- 模式
選好要縮排的行之後按大於 ( > )
被選到的行就會縮排囉
2. 單行縮排
在一般模式下
游標在該行上,按兩下大於 ( >> )
那一行就會縮排了
3. 將大括弧的整個區塊縮排
先將游標移到括弧上,
按下大於,百分比 ( >% )
整個括弧區塊就會一起縮排囉
參考
2019年1月19日 星期六
Gaussian
Gaussian softwarehttps://www.youtube.com/playlist?list=PL3T-qmaDVsdD2ZVH1aAMusQrpNLJZllbD
CO2
https://www.youtube.com/watch?v=WkzxdhCtsQQ
CH3COOH
https://www.youtube.com/watch?v=RIQCL0oIeus
設定旋轉角,製作script檔以及光譜分析
https://www.youtube.com/watch?v=KSGg-PmJt4E
CO2
https://www.youtube.com/watch?v=WkzxdhCtsQQ
CH3COOH
https://www.youtube.com/watch?v=RIQCL0oIeus
設定旋轉角,製作script檔以及光譜分析
https://www.youtube.com/watch?v=KSGg-PmJt4E
2019年1月15日 星期二
華碩主板禁用UEFI安全啟動(Disable Secure Boot for ASUS Motherboard)
以下disable掉安全啟動的方法:
1.按F2/ESC進入BIOS
2.確定 "OS Type" 是 "Windows UEFI"
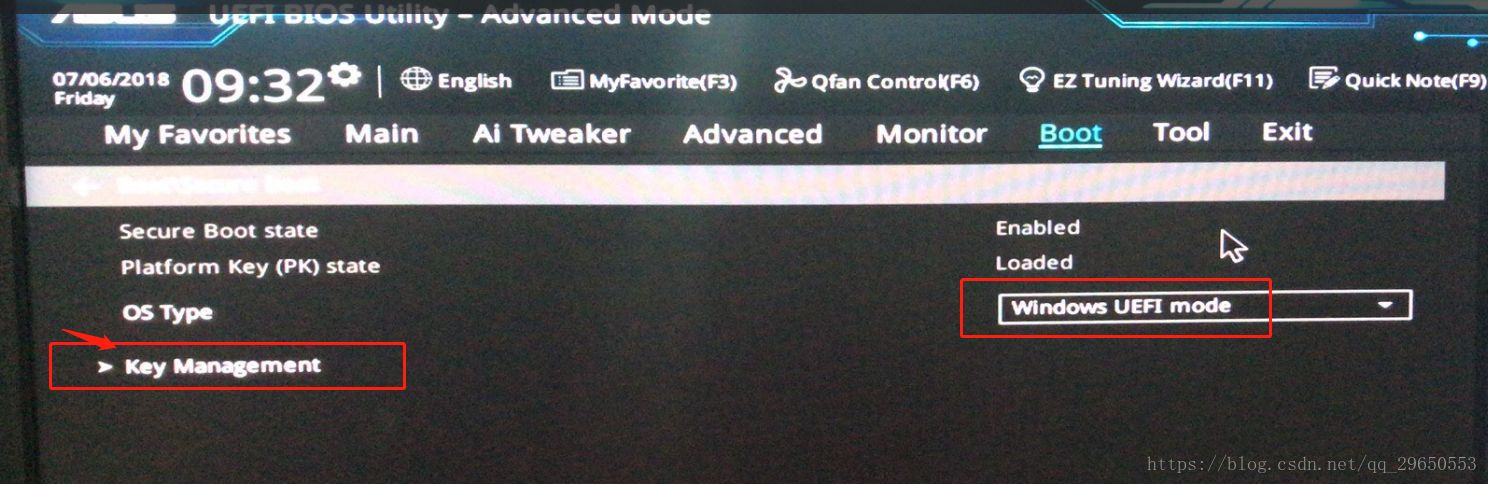
3.點擊進入 "Key Management"
以下每一項上面點右鍵——"Clear Secure Boot keys"
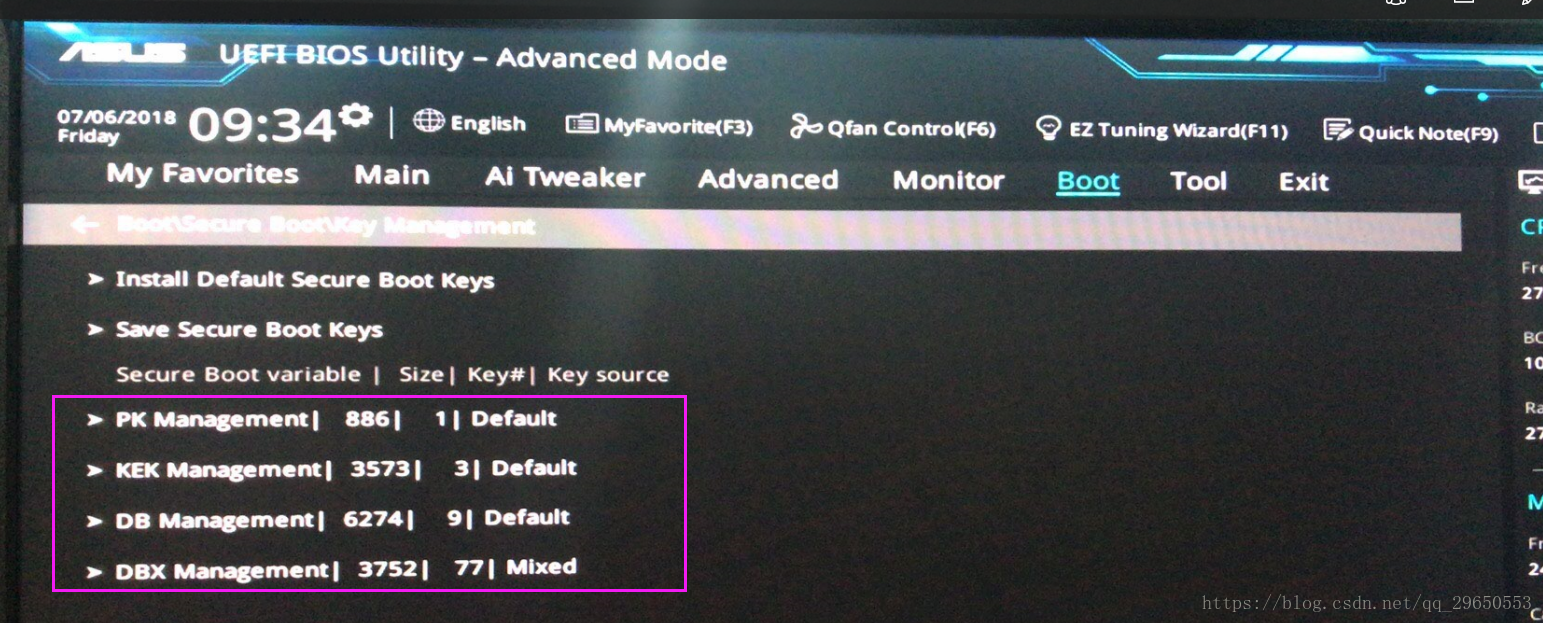
4. 刪除完成後,Secure Boot state 將自動變為 disabled,然後設置OS Type 為 Other OS .
1.按F2/ESC進入BIOS
2.確定 "OS Type" 是 "Windows UEFI"
3.點擊進入 "Key Management"
以下每一項上面點右鍵——"Clear Secure Boot keys"
4. 刪除完成後,Secure Boot state 將自動變為 disabled,然後設置OS Type 為 Other OS .
參考
2019年1月12日 星期六
Amber force field單位轉換到Gromacs
For non-bonded:
- εgro=εGAFF·4.184
- σgro=RGAFF·25/6 * 1/10 = 0.17818·RGAFF
For bonded:
- Bonds: b0gro = b0GAFF / 10 ; kb,gro = kb,GAFF /10 *2 *4.184
- Angles: Egro = Emin/2 cth = EGAFF *2 * 4.184
- Dihedrals: kdgro = PKGAFF * 4.184 / IDIVFGAFF
參考
https://gcm.upc.edu/en/members/luis-carlos/molecular-dynamicshttps://mailman-1.sys.kth.se/pipermail/gromacs.org_gmx-users/2015-September/101115.html
https://github.com/lanl/gromacs-kokkos/blob/master/src/gromacs/math/units.h
2019年1月8日 星期二
安裝Lammps polarizable systems using Drude oscillators套件
ERROR: Illegal pair_style command (../pair_lj_cut_coul_long.cpp:600)
Last command: pair_style hybrid/overlay lj/cut/coul/long 8.0 8.0 thole 2.600 8.0
$ make yes-USER-DRUDE
ERROR: Unknown fix style shake (../modify.cpp:898)
Last command: fix fSHAKE gATOMS shake 0.0001 20 0 b 2 3 5
$ make yes-RIGID
Last command: pair_style hybrid/overlay lj/cut/coul/long 8.0 8.0 thole 2.600 8.0
$ make yes-USER-DRUDE
ERROR: Unknown fix style shake (../modify.cpp:898)
Last command: fix fSHAKE gATOMS shake 0.0001 20 0 b 2 3 5
$ make yes-RIGID
2019年1月5日 星期六
OpenMM
Introduction to Running Simulations with OpenMM
http://videominecraft.ru/watch/hbQ_dfgWPc8/introduction-to-running-simulations-with-openmm.html
Free Energy Calculations and OpenMM
http://videominecraft.ru/watch/hbQ_dfgWPc8/introduction-to-running-simulations-with-openmm.html
Free Energy Calculations and OpenMM
MS
An Introduction to Materials Studiohttp://videominecraft.ru/watch/c1klTPfhJV8/an-introduction-to-materials-studio.html
Materials Studio Tutorial 1: Introduction, basic structure construction and view options
DFT calculations with Materials Studio (sigma profile/cosmo theory)
Materials Studio Tutorial 1: Introduction, basic structure construction and view options
Ovito
Introduction to Ovito
OVITO Modifiers for Crystal Structures
Using Ovito to Analyze a LAMMPS Simulation for Dislocation Velocity
2019年1月4日 星期五
2019年1月3日 星期四
Ubuntu 18.04 安裝NVIDIA驅動程式
ubuntu18.04安裝NVIDIA顯卡驅動有兩種方式,一種是用ubuntu的apt,另一種是NVIDIA官網下載的驅動程式,以下是兩種驅動安裝方式:
安裝/移除kernel的方法
這邊紀錄一下安裝/移除Linux kernel的方法,因為有時候更新kernel可以達到讓週邊硬體獲得更好的使用,及獲得更好的執行效率和效能。(例如:wireless連線速率改善、USB外接硬碟連接問題(有時候無法連接存取)改善...) 但也有可能安裝失敗,或是有多餘舊的kernel不想保留,而想要將他們移除。
2019年1月2日 星期三
Thread與Warp
__ballot(int predicate):指的是當前線程所在的Wrap中第N個線程對應的predicate值不爲零,則將整數零的第N位進行置位
__popc(ballot(int predicate)):返回warp中bool不爲零的線程數目
asm("mov.u32 %0, %laneid;" : "=r"(ret)):獲得ret爲當前線程在所在Warp中的ID
%lanemask_lt:32-bit mask with bits set in positions less than the thread's lane number in the warp
https://docs.nvidia.com/cuda/parallel-thread-execution/index.html.
__popc(ret & __ballot(int predicate)):返回的值爲當前線程在所在的Warp中是第幾個滿足條件的
__popc ( unsigned int x ): Count the number of bits that are set to 1 in x.
https://docs.nvidia.com/cuda/cuda-math-api/group__CUDA__MATH__INTRINSIC__INT.html
__popc(ballot(int predicate)):返回warp中bool不爲零的線程數目
asm("mov.u32 %0, %laneid;" : "=r"(ret)):獲得ret爲當前線程在所在Warp中的ID
%lanemask_lt:32-bit mask with bits set in positions less than the thread's lane number in the warp
https://docs.nvidia.com/cuda/parallel-thread-execution/index.html.
__popc(ret & __ballot(int predicate)):返回的值爲當前線程在所在的Warp中是第幾個滿足條件的
__popc ( unsigned int x ): Count the number of bits that are set to 1 in x.
https://docs.nvidia.com/cuda/cuda-math-api/group__CUDA__MATH__INTRINSIC__INT.html
Example:
nvcc -arch=sm_61 -o popc popc.cu
由於__ballot(x > 10),所以__popc(a[x])會累計32個thread邏輯成立,__popc(b[x] & a[x])是當前線程在所在的Warp中是第幾個滿足條件
參考
#include <iostream>
#include <cstdio>
using namespace std;
__device__ __forceinline__ int laneId()
{
unsigned int ret;
asm("mov.u32 %0, %laneid;" : "=r"(ret));
return ret;
}
__device__ __forceinline__ int laneMaskLt()
{
unsigned int ret;
asm("mov.u32 %0, %lanemask_lt;" : "=r"(ret));
return ret;
}
__global__ void testKernel(int *a, int *b, int *c, int *d, int *e, int n)
{
int x = threadIdx.x + blockIdx.x * blockDim.x;
if (x >= n)
{
return;
}
a[x] = __ballot(x > 10);
b[x] = laneMaskLt();
d[x] = __popc(b[x] & a[x]);
c[x] = __popc(a[x]);
e[x] = laneId();
}
int main()
{
int *a, *b, *c, *d, *e, *dev_a, *dev_b, *dev_c, *dev_d, *dev_e;
int n = 64;
int size = n * sizeof(int);
a = (int *)malloc(size);
b = (int *)malloc(size);
c = (int *)malloc(size);
d = (int *)malloc(size);
e = (int *)malloc(size);
cudaMalloc(&dev_a, size);
cudaMalloc(&dev_b, size);
cudaMalloc(&dev_c, size);
cudaMalloc(&dev_d, size);
cudaMalloc(&dev_e, size);
testKernel<<<1, n>>>(dev_a, dev_b, dev_c, dev_d, dev_e, n);
cudaMemcpy(a, dev_a, size, cudaMemcpyDeviceToHost);
cudaMemcpy(b, dev_b, size, cudaMemcpyDeviceToHost);
cudaMemcpy(c, dev_c, size, cudaMemcpyDeviceToHost);
cudaMemcpy(d, dev_d, size, cudaMemcpyDeviceToHost);
cudaMemcpy(e, dev_e, size, cudaMemcpyDeviceToHost);
for (int i = 0; i < n; ++i)
{
printf("%d %d %d %d %d\n", a[i], b[i], c[i], d[i], e[i]);
}
cudaFree(dev_a);
cudaFree(dev_b);
cudaFree(dev_c);
cudaFree(dev_d);
cudaFree(dev_e);
free(a);
free(b);
free(c);
free(d);
free(e);
}
n=64代表這64個thread會被分成兩個Warp ,第一個Warp會印出由於__ballot(x > 10),所以__popc(a[x])會累計32個thread邏輯成立,__popc(b[x] & a[x])是當前線程在所在的Warp中是第幾個滿足條件
2019年1月1日 星期二
利用TPPMKTOP手動找force-field
TPPMKTOP是一種快速搜尋force-field的網頁工具,可以產生opls型式的itp檔讓分子模擬套裝軟體直接快速地讀取force-field,不必花費大量的時間找尋每個原子的Lennard-jones位能以及原子間的bond鍵能和鍵長,但如果想要知道這些資料細節,就得從itp檔近一步去分析,以下內容就是介紹如何從TPPMKTOP產生itp檔去找相對應的分子資訊。
Accelerated Computing
1. Introduction
An Even Easier Introduction to CUDA (20170125)
https://devblogs.nvidia.com/even-easier-introduction-cuda/
CUDA從入門到精通
https://blog.csdn.net/Augusdi/article/details/12833235
GPUS Ladyhttps://cloud.tencent.com/developer/user/1539448
An Even Easier Introduction to CUDA (20170125)
https://devblogs.nvidia.com/even-easier-introduction-cuda/
CUDA從入門到精通
https://blog.csdn.net/Augusdi/article/details/12833235
GPUS Ladyhttps://cloud.tencent.com/developer/user/1539448
CUDA Pro Tip: Optimized Filtering with Warp-Aggregated Atomics (20141001)
https://devblogs.nvidia.com/cuda-pro-tip-optimized-filtering-warp-aggregated-atomics/
GPU Pro Tip: Fast Histograms Using Shared Atomics on Maxwell (20150317)
Accelerating Dissipative Particle Dynamics Simulation on Tesla GPUs (20150416)
https://devblogs.nvidia.com/accelerating-dissipative-particle-dynamics-simulation-tesla-gpus/
Voting and Shuffling to Optimize Atomic Operations (20150806)
__shfl_down and __shfl_down_sync give different results
Using CUDA Warp-Level Primitives (20180115)
instruction
https://docs.nvidia.com/cuda/cuda-c-programming-guide/index.html#warp-vote-functions
DPD slides
http://on-demand.gputechconf.com/gtc/2014/presentations/S4518-dissipative-particle-dymanics-sims-kepler.pdf
warp shuffles, and reduction / scan operations
DPD slides
http://on-demand.gputechconf.com/gtc/2014/presentations/S4518-dissipative-particle-dymanics-sims-kepler.pdf
warp shuffles, and reduction / scan operations
閱讀Warp Vote Functions
閱讀Warp Shuffle Functions
CUDA之Warp Shuffle詳解
cuda的Shuffle技術以及自定義雙精度版本
3. Unified Memory
Beyond GPU Memory Limits with Unified Memory on Pascal (20161214)
Beyond GPU Memory Limits with Unified Memory on Pascal (20161214)
https://devblogs.nvidia.com/beyond-gpu-memory-limits-unified-memory-pascal/
Unified Memory for CUDA Beginners (20170619)
Maximizing Unified Memory Performance in CUDA (20171119)
https://devblogs.nvidia.com/maximizing-unified-memory-performance-cuda/
4. C++11
The Power of C++11 in CUDA 7 (20150318)https://devblogs.nvidia.com/power-cpp11-cuda-7/
C++11 in CUDA: Variadic Templates (20150326)
Unified Memory for CUDA Beginners (20170619)
Maximizing Unified Memory Performance in CUDA (20171119)
https://devblogs.nvidia.com/maximizing-unified-memory-performance-cuda/
4. C++11
The Power of C++11 in CUDA 7 (20150318)https://devblogs.nvidia.com/power-cpp11-cuda-7/
C++11 in CUDA: Variadic Templates (20150326)
CUDA 7 Release Candidate Feature Overview: C++11, New Libraries, and More (20150113)
https://devblogs.nvidia.com/cuda-7-release-candidate-feature-overview/
5. COOPERATIVE GROUPS
Cooperative Groups: Flexible CUDA Thread Programming (20171004)
未來會增加
Streams, zero-copy memory, texture objects, PTX (parallel thread execution) assembly, warp-level vote/shuffle
5. COOPERATIVE GROUPS
Cooperative Groups: Flexible CUDA Thread Programming (20171004)
https://devblogs.nvidia.com/cooperative-groups/
CUDA 9 AND BEYOND
https://drive.google.com/file/d/1YipovGErr3mfCBG3dqlaAgfUcFt__BCd/view?usp=sharing
Cooperative Groups
https://drive.google.com/file/d/13eN5flsds307eIIAtwBNAq8BScscoyOe/view?usp=sharing
6. Stream
https://devblogs.nvidia.com/how-overlap-data-transfers-cuda-cc/
CUDA 9 AND BEYOND
https://drive.google.com/file/d/1YipovGErr3mfCBG3dqlaAgfUcFt__BCd/view?usp=sharing
Cooperative Groups
https://drive.google.com/file/d/13eN5flsds307eIIAtwBNAq8BScscoyOe/view?usp=sharing
6. Stream
https://devblogs.nvidia.com/how-overlap-data-transfers-cuda-cc/
7. Other
CUDA Spotlight: Michela Taufer on GPU-Accelerated Scientific Computing (20140821)https://devblogs.nvidia.com/cuda-spotlight-michela-taufer-gpu-accelerated-scientific-computing/
CUDA Spotlight: Michela Taufer on GPU-Accelerated Scientific Computing (20140821)https://devblogs.nvidia.com/cuda-spotlight-michela-taufer-gpu-accelerated-scientific-computing/
Register Cache: Caching for Warp-Centric CUDA Programs (20171012)
未來會增加
Streams, zero-copy memory, texture objects, PTX (parallel thread execution) assembly, warp-level vote/shuffle
訂閱:
文章 (Atom)