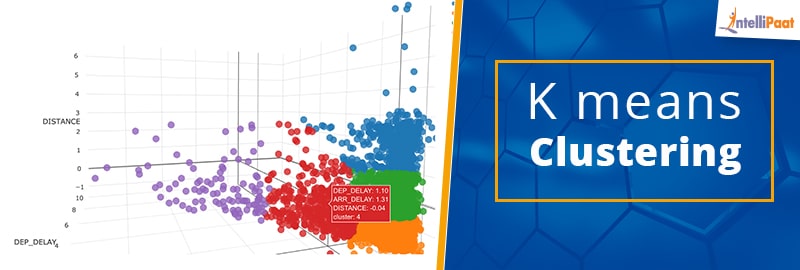
Introduction to K means Clustering in Python
K means clustering演算法是一種非常常見的無監督學習演算法。 該演算法將n個對象分群為k個clusters,其中每個對象具有最近均值的cluster。
這是該模組的表格:
- What Is Clustering Algorithm?
- Types of Clustering Algorithm
- What Is K means Clustering Algorithm?
- K means Clustering Algorithm Using Sklearn in Python- Iris Dataset
Without much delay, let’s get started.
What Is Clustering Algorithms?Clustering只不過是將一組數據劃分為相似點或特徵的組,其中同一組中的數據點盡可能相似,不同組中的數據點盡可能不同。 我們在日常生活中使用clustering; 例如,在超市中,所有蔬菜被分組為一組,所有水果被分組為另一組。 這種clustering可以幫助客戶加快購物流程。

我們可能遇到的另一個clustering範例是Amazon或Flipkart產品推薦。 Amazon或Flipkart根據我們之前的搜索推薦我們的產品。 他們是如何做到的呢? 嗯,這背後的概念是clustering。
現在我們知道了什麼是clustering,讓我們討論一下clustering中的種類。
Types of Clustering Algorithms
有三種主要類型的clustering技術,它們如下:
Exclusive clustering:在獨占聚類中,數據以獨占方式分組,以便某個數據只屬於一個確定的cluster。

Overlapping clustering:在重疊聚類中,每個點可以屬於兩個或更多個clusters。

Hierarchical clustering:在此技術中,第一步是分配自己的所有數據點clusters。 第二步是將兩個較近的clusters合併為一個cluster。 第三步是計算新cluster與每個舊clusters之間的距離。 再次,重複第二步和第三步,直到只剩下一個cluster。

好了,現在我們知道了clustering的類型,讓我們繼續討論真正的話題,K means clustering。
What Is K means Clustering Algorithm?
K means clustering是一種演算法,其主要目標是將類似的數據點分組到一個cluster中。 在K means clustering,k表示組或clusters的總數。 K表示clustering在歐幾里德距離計算上運行。 現在,讓我們理解K means clustering如何進行。
比如,我們有一個由10名球員的身高和體重訊息組成的數據集。 我們需要根據它們的身高和體重將它們分成兩組。
第1步:初始化cluster質心
步驟2:計算從每個觀測到初始clusters的歐幾里德距離

第3步:找到新的cluster質心
新Cluster 1 =(182.6,72.6)
新Cluster 1 =(170.4,89.2)
步驟4:再次,計算歐幾里德距離
計算從每個觀察到Cluster 1和Cluster 2的歐幾里德距離
重複步驟2,3和4,直到cluster中心不再更改為止
現在,讓我們看下面的實作,以便更深入地了解K-means演算法。
Hands-on: K-means Clustering Algorithm Using Sklearn in Python- Iris Dataset
數據集
我們將使用Edgar Anderson在20世紀30年代收集的著名的Iris數據集。 在這個例子中,我們將訓練一個隨機森林分類算法來預測測試數據中的類別。

讓我們看看在這個問題陳述中我們如何使用Python執行K means clustering。 為了執行K-means,我們將在Python中使用sklearn函式庫。 讓我們開始吧。
第1步:加載Iris數據集

第2步:看一下形狀

第3步:瀏覽一下這些特徵


第4步:瀏覽一下目標


第5步:構建模型

第6步:設置clusters數量

第7步:將特徵安裝到模型中

第8步:預測並標記數據






參考
https://intellipaat.com/blog/k-means-clustering/#What-Is-Clustering
沒有留言:
張貼留言